Introduction
In an era defined by rapid digital transformation and an overwhelming influx of data, organizations face the challenge of effectively managing and utilizing their vast information resources. As businesses strive to stay competitive, the role of data science has become increasingly critical.
This blog explores how data science serves as a powerful tool to unlock the potential within an organization’s data portfolio management and how it enhances Enterprise Architecture (EA). We will delve into the ways data science refines data management, drives strategic decision-making, and aligns IT infrastructure with business goals, ultimately positioning organizations for success in a data-driven world.
What is Data Science?
The primary goal of data science is to convert raw data into actionable insights that can drive strategic decision-making, enhance operational efficiency, drive innovation and foster growth.
Data science involves several key steps:
- data collection
- data cleaning and
- data analysis
During the collection phase, data is gathered from diverse sources, ensuring it is comprehensive and relevant. Next step, data cleaning, involves addressing inconsistencies, missing values, and errors to prepare the data for analysis. Finally, through data analysis, patterns, trends, and correlations are identified, which can be used to make informed predictions and decisions. Techniques such as machine learning and natural language processing further enhance the depth and accuracy of these insights, allowing organizations to address challenges, optimize processes, and create substantial value.
Enhance Your Data Portfolio with Data Science
Every organization has a data portfolio – a collection of data gathered over time. It includes structured data, such as databases and spreadsheets, as well as unstructured data such as emails, documents and multimedia files. This portfolio reflects the breadth and depth of the organization’s information resources, accumulated from various activities, transactions, and interactions. Properly managing the data portfolio is crucial as it enables organizations to leverage their data for analytics, reporting, and decision-making.
Data science can significantly enhance a data portfolio by applying advanced techniques to maximize the value from accumulated data. By leveraging elements of statistical analysis, machine learning, and computer science, data science processes and analyses both structured and unstructured data within the portfolio to extract meaningful insights. This involves several key areas of focus:
Data Collection Optimization
Data science can improve the data collection phase by identifying the most relevant and valuable data sources, ensuring that the gathered data is comprehensive and pertinent. This enhances the overall quality and relevance of the data portfolio.
Data Cleaning Efficiency
Through automated tools and algorithms, data science streamlines the data cleaning process, effectively addressing inconsistencies, missing values, and errors. This ensures that the data is accurate and reliable, which is crucial for subsequent analysis and decision-making.
Advanced Data Analysis
Data science employs sophisticated techniques such as machine learning, natural language processing, and predictive analytics to analyse data. These techniques help uncover patterns, trends, and correlations within the data portfolio that might not be evident through traditional analysis methods.
Actionable Insights
By transforming raw data into actionable insights, data science enables organizations to make informed decisions based on real-time and historical data. This can drive strategic decision-making and provide a competitive advantage.
Predictive Capabilities
Data science can enhance a data portfolio by developing predictive models that forecast future trends and behaviors. This allows organizations to anticipate challenges, optimize processes, and proactively respond to market changes.
Resource Optimization
By analysing data on resource usage and operational efficiency, data science helps organizations streamline their operations, reduce costs, and improve productivity.
Strategic Support
A well-analysed data portfolio supports strategic initiatives by providing a solid foundation of evidence-based insights. This aids in formulating effective strategies and achieving organizational goals.
To get deeper insights, it’s essential to analyze a variety of data sources, including internal systems such as CRM and ERP databases, operational logs, and external sources like market research reports and social media data. By analyzing these sources, organizations can extract insights, such as customer behavior patterns, operational efficiencies, and market trends. Data science can then be applied to enhance these sources further. For instance, advanced analytics might reveal hidden correlations in transaction data, predictive models could improve demand forecasting, and natural language processing might extract sentiment from social media feeds. Such thorough examination not only identifies gaps and inconsistencies but also leads to the creation of new data products that drive actionable intelligence and strategic decision-making.
Why is Data Science Important for Enterprise Architecture?
Data science is integral to Enterprise Architecture (EA), which aims to align an organization’s IT systems with its strategic goals. As the digital landscape evolves rapidly, data science delivers the analytical tools and insights required to refine and advance EA, ensuring that IT infrastructure remains agile and responsive to changing business needs.
One of the primary contributions of data science to EA is its ability to analyse complex datasets to pinpoint inefficiencies and opportunities within IT systems. By examining historical data related to system performance, user interactions, and operational workflows, data stewards can identify patterns that reveal areas where the architecture may be lacking or misaligned with business objectives. For instance, data analysis might uncover redundancies in system integrations or inefficiencies in workflow processes, enabling targeted improvements that enhance overall system efficiency and performance.
Data science also facilitates predictive analytics, which is crucial for proactive decision-making in EA. Advanced machine learning models can forecast future trends and needs, such as anticipated spikes in user demand or potential system failures. This foresight allows organizations to anticipate changes and adapt their IT infrastructure accordingly, ensuring that the architecture remains scalable, resilient, and capable of meeting future demands.
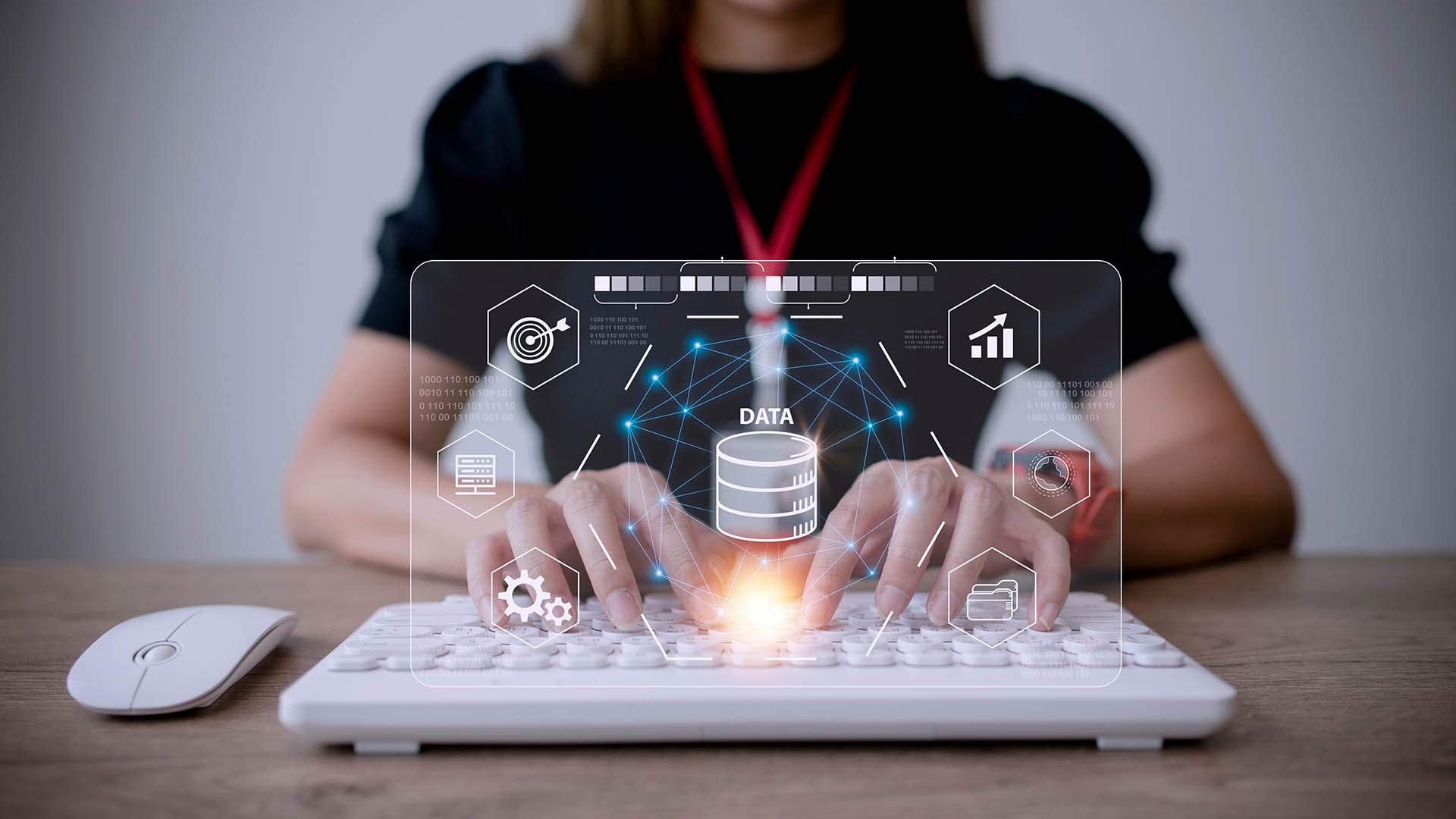
In addition to optimizing current systems, data science also supports the evaluation of new technologies and architectural changes. By providing sophisticated analytical tools, data science enables organizations to measure the impact of new systems, processes, or technologies on business performance. This data-driven approach ensures that architectural modifications are achieving their intended outcomes and contributing to business objectives, promoting a more agile and responsive IT environment.
Moreover, significant application of data science within enterprise architecture is the evaluation and management of application components. Data science can be used to develop key performance indicators (KPIs) for assessing applications, such as usage patterns and customer satisfaction. By analysing these KPIs, organizations can identify underused applications, which might lead to cost savings through decommissioning, and uncover applications with frequent errors that indicate quality issues. Techniques like box-in-box charts and heatmaps can visualize these results, providing immediate insights into application performance and usage. This visualization aids in Application Portfolio Management (APM) by supporting data-driven decisions about which applications to retain, optimize, or phase out. .
Finally, data science enhances decision-making by integrating and analysing data from multiple sources, such as financial metrics, operational data, and customer feedback. This holistic view allows enterprise architects to understand how different components of the IT infrastructure interact and contribute to overarching business goals. Consequently, it leads to well-informed decisions regarding technology investments and resource allocation, aligning IT capabilities with business needs.
How EA Tools Enhance Data Portfolio Management and Data Science
A structured and effective EA relies on comprehensive tools that adhere to standards like ArchiMate, which offer a clear framework for modelling and visualizing an organization’s IT landscape and processes. EA tools are essential for managing the complexity of data portfolios and ensuring alignment between technology and business strategy. They facilitate a comprehensive understanding of data flows and dependencies through data mapping and relationship modelling. This visibility is crucial for applying data science effectively, as it helps identify key data sources and potential gaps in the data portfolio. Additionally, these tools support data governance by ensuring data quality and consistency, which is essential for accurate analysis. They also provide the infrastructure and integration points necessary for implementing advanced analytics and machine learning models. With this integration, organizations can derive valuable insights from their data, optimize IT resources, and align technology strategies with business objectives. In essence, data science, when integrated with effective EA tools, enhances the evaluation and refinement of the application portfolio, ensuring it aligns with strategic goals and supports overall organizational efficiency.
Summary
Data science and Enterprise Architecture (EA) together play a critical role in optimizing how organizations manage and leverage their data portfolios. By refining data collection, cleaning, and analysis, data science transforms raw data into actionable insights, supporting strategic decision-making and operational efficiency. Leveraging an EA tool, such as ADOIT, provides a structured framework for modeling and analysis, enhancing the ability to identify inefficiencies, support predictive analytics, and align IT infrastructure with business goals. This integration is essential for driving growth and maintaining a competitive advantage in a rapidly evolving digital landscape.